Abstract Article Google Scholar Introduction In this study, models constructed for both male and female players demonstrated good performance, highlighting the importance of technical action frequency in capturing the tactical styles of badminton athletes. Notably, the model based on male player data exhibited superior performance across various metrics, suggesting fundamental differences in tactical styles between […]
Abstract
Introduction
Methods
Data collection
Wang, W.-Y., Shuai, H.-H., Chang, K.-S. & Peng, W.-C. ShuttleNet position-aware fusion of rally progress and player styles for stroke forecasting in badminton. Proc. AAAI Conf. Artif. Intell. 36(4), 4217–4229 (2022).Article
Google Scholar
Preprocessing
Article
CAS
PubMed
PubMed Central
Google Scholar
Model description
Article
CAS
PubMed
MATH
Google Scholar
Website construction and availability
Song, S. et al. Deep reinforcement learning for modeling human locomotion control in neuromechanical simulation. J. Neuroeng. Rehabil. 18 (1), 126 (2021).Wang, J., Keusters, W. R., Wen, L. & Leeflang, M. M. G. IPDmada: An R Shiny tool for analyzing and visualizing individual patient data meta-analyses of diagnostic test accuracy. Res. Synthesis Methods. 12(1), 45–54 (2021).
Results
Identification of critical technical movements contributing to success in competitions
The data, divided by sex, years, and competition outcome, was split into a training set and a test set with a ratio of 7:3. Specifically, the training set for men’s competitions contained 250 samples, while the test set contained 108 samples; the women’s competitions training set included 240 samples, and the test set contained 104 samples. This data division method ensured sufficient model training and reliable test results (Fig. 1).Article
MATH
Google Scholar
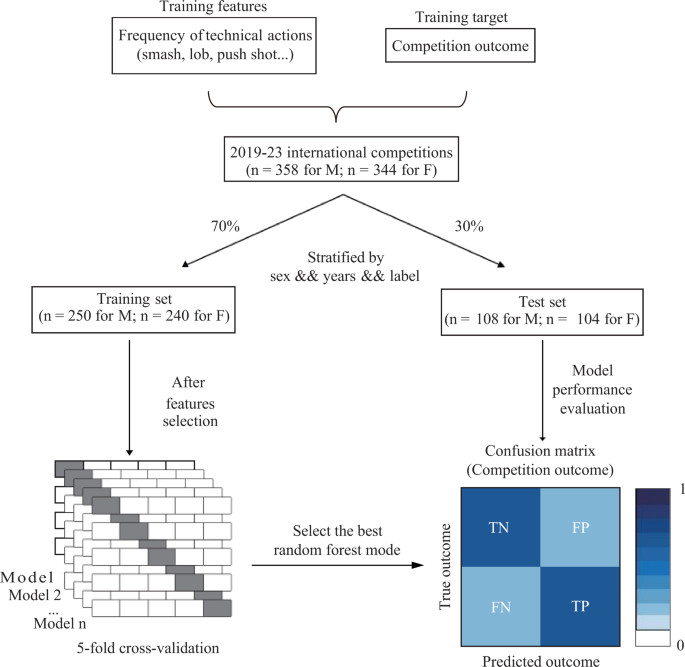
Article
PubMed
MATH
Google Scholar
Quantitative analysis and model performance validation of the impact of key technical actions on badminton competition outcomes
Article
CAS
PubMed
PubMed Central
MATH
Google Scholar
Reprints and permissions
Deciphering victory factors: an interactive web application for analyzing badminton matches
The authors declare no competing interests.
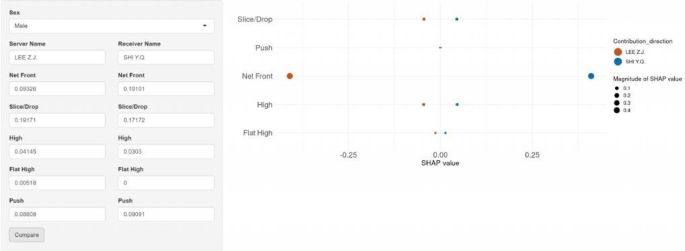
Discussion
Google Scholar
In the development of our interactive web platform, we employed the Shiny framework from the R programming environment24,25. Shiny enables the creation of interactive web applications, allowing for the integration of analytical computations with visualization capabilities15,26. Designed for intuitive use, the platform facilitates player comparisons and predictive analytics in badminton, with Shiny modules enabling data processing and visualization. The statistical models and analytical methods are grounded in R, ensuring data analysis rigor and result reliability. This platform demonstrates the effective use of R and Shiny in sports analytics.Article
MathSciNet
MATH
Google Scholar
Conclusion
Data availability
Phomsoupha, M. & Laffaye, G. The science of badminton: Game characteristics, anthropometry, physiology, visual fitness and biomechanics. Sports Med. (Auckland NZ). 45(4), 473–495 (2015).
References
- Badminton, celebrated for its fast-paced and competitive nature, enjoys global popularity1. However, the complexity of factors influencing match outcomes presents a significant challenge for researchers, coaches, and athletes2. Existing studies have explored these determinants from various perspectives, such as athletes’ physical conditions, technical skills, psychological states, and physiological tests3,4,5,6. Despite these efforts, accurately predicting match outcomes remains a daunting task due to inherent uncertainties in gameplay dynamics7.PubMed Google Scholar
- Qing YiAfter the model was constructed, to further understand the specific impact of each feature on the outcomes of competitions, we calculated the SHAP values for each feature. The analysis showed that among the top five key features evaluated, the technical actions of ‘Net Front’, ‘Slice/Drop’, and ‘Push’ had a significant impact on the outcomes of both men’s and women’s competitions, presenting a consistent pattern. Particularly for ‘Net Front’, its SHAP values increased with the value, indicating that a higher frequency of this technical action by the serving side compared to the receiving side is more likely to lead to victory in the competition. Conversely, the situations for ‘Slice/Drop’ and ‘Push’ were opposite (Fig. 2C,D).
- Chen, Y., Zulnaidi, H. & Syed Ali, S. K. B. Study on the eye movement characteristics of the badminton practitioners of different levels regarding visual attention. Front. Psychol. 13, 1026006 (2022).Article
PubMed
Google Scholar - Ooi, C. H. et al. Physiological characteristics of elite and sub-elite badminton players. J. Sports Sci. 27(14), 1591–1599 (2009).PubMed Google Scholar
- Significant advancements in machine learning have further expanded the scope of badminton technical and tactical analysis. Previous studies have explored challenges such as motion recognition, tactical pattern mining, and match outcome prediction10. For example, research on An Se-young successfully established a scoring and losing prediction model using an improved data classification method, achieving a prediction accuracy of 87.5% with support vector machines11. Another study proposed a deep reinforcement learning-based approach that evaluates tactical behaviors using Q-functions, offering more detailed insights into players’ actions12. Furthermore, the development of a 3D visualization system, TIVEE, has provided a multi-level exploration tool for analyzing spatial dynamics of tactics13, while drone-based datasets have facilitated team coordination analysis in doubles matches through control area probability distributions14. These advancements underscore the potential of machine learning techniques in uncovering valuable insights into technical and tactical aspects of badminton, yet further exploration is needed to enhance their predictive precision and practical application.Article
ADS
CAS
PubMed
PubMed Central
MATH
Google Scholar - Overview of study.
- School of Kinesiology, Shanghai University of Sport, Qingyuanhuan Road, #650, Yangpu District, Shanghai, ChinaAI for Science, Shanghai Artificial Intelligence Laboratory, Shanghai, China
- Ding, N., Takeda, K., Jin, W., Bei, Y. & Fujii, K. Estimation of control area in badminton doubles with pose information from top and back view drone videos. Multimedia Tools Appl. 83(8), 24777–24793 (2023).Vitorio, R., Mancini, M., Carlson-Kuhta, P., Horak, F. B. & Shah, V. V. Should we use both clinical and mobility measures to identify fallers in Parkinson’s disease? Parkinsonism Relat. Disord. 106, 105235 (2023).
- Naughton, M., Weaving, D., Scott, T. & Compton, H. Synthetic data as a strategy to resolve data privacy and confidentiality concerns in the Sport sciences: practical examples and an R Shiny application. Int. J. Sports Physiol. Perform. 18 (10), 1213–1218 (2023).
- Article
PubMed
PubMed Central
MATH
Google Scholar - PubMed Google ScholarRubio-Morales, A., Díaz-García, J., Harper, L. D. & García-Calvo, T. The influence of the perceived requirements of the next match and motivation on the mental fatigue of soccer players. Scand. J. Med. Sci. Sports. 34 (2), e14580 (2024).
- Accepted: Gomez, M. A., Leicht, A. S., Rivas, F. & Furley, P. Long rallies and next rally performances in elite men’s and women’s badminton. PloS One. 15 (3), e0229604 (2020).
- Download referencesAnyone you share the following link with will be able to read this content:
- Yuan, H., Wang, Y., Yang, K. & Bin, Y. Prediction model and technical and tactical decision analysis of women’s badminton singles based on machine learning. PloS One. 19(11), e0312801 (2024).Roberts, C. Modelling patterns of agreement for nominal scales. Stat. Med. 27(6), 810–830 (2008).
- The application of machine learning techniques to predict badminton match outcomes through the analysis of technical actions seems to represent an area that has not yet been extensively investigated within the existing body of research. This study aims to interpret this phenomenon by developing a predictive model based on the frequency of technical actions, utilizing machine learning techniques. Focusing on international competitions from 2019 to 2023, we collected data on 23 distinct technical actions (e.g., Net Front, Slice/Drop, Push) to construct predictive models. The study distinguishes itself by employing a Random Forest algorithm to ascertain the significance of each technical action, utilizing forward stepwise selection and 5-fold cross-validation for feature refinement. SHAP value analysis further validated the pivotal roles of ‘Net Front’, ‘Slice/Drop’, and ‘Push’ across both sexes, linking higher frequencies of ‘Net Front’ with increased match-winning probabilities. Model validation on a test set demonstrated effective performance in both sexes, with the model based on male data exhibiting higher accuracy and predictive values, surpassing the performance of the female data model. This comprehensive examination, grounded in quantitative analysis, not only enhances our understanding of badminton gameplay dynamics but also offers valuable insights for coaching strategies and training methodologies. To extend the applicability of our findings and facilitate user engagement, we developed a web application based on our model. This platform enables players, coaches, and researchers to input player characteristics and receive strategic recommendation through an intuitive interface, further leveraging the machine learning model’s capabilities to support tactical decision-making before badminton competitions.Interactive Web Application Display: Comparative Analysis of Technical Action Frequencies – Lee Zii Jia vs. Shi Yuqi.
- The study employed video observation methods to statistically analyze technical actions and match outcomes5,15. Four badminton players holding a second-level athlete classification from the research group, each with over six months of experience, were selected as data collectors. These collectors uploaded the recorded videos to the “Match Analysis” application, a software for analyzing badminton skills and tactics developed in collaboration with Shanghai Danzhu Sports Technology and Culture Co., Ltd. Subsequently, the four members independently observed and recorded statistics from the videos. To ensure the accuracy and reliability of the data, both inter-rater and intra-rater consistency tests were conducted. The data collected during the initial session by the four individuals were evaluated using the Intraclass Correlation Coefficient (ICC), with an ICC value of 0.94 (p < 0.01), indicating high consistency among the raters. For the intra-rater reliability test, Cohen’s Kappa coefficient was utilized, which is commonly used for assessing agreement between two raters for categorical variables16. The Kappa coefficient was 0.96 (p < 0.01), demonstrating a high degree of consistency in the data17.Article
MathSciNet
PubMed
MATH
Google Scholar - Article
PubMed
Google Scholar
Article
Google Scholar - Sheng, Y., Liu, C., Yi, Q. et al. Predicting badminton outcomes through machine learning and technical action frequencies.
Sci Rep 15, 10575 (2025). https://doi.org/10.1038/s41598-025-87610-7Download citation - Li, Q. & Ding, H. Construction of the structural equation model of badminton players’ variable direction ability and its enlightenment to sports training. Annals Palliat. Med. 10(4), 4623–4631 (2021).Published:
- After collecting the data, we first conducted a statistical analysis of the types of technical actions in all matches, identifying a total of 23 initial features (High, Smash, Dribble, Push, Slice/Drop, Lift, Block Smash, Net Front, Clear, Net Drop from Lift, Lift from Slice, Pull, Hook, Slice Lift, Block, Lift Smash, Block Hook, Hook from Lift, Drive, Net Drop from Slice Drive, Lift from Slice Drive, Flat High, and Block from Slice Drive). Then, we calculated the frequency of technical actions for both the serving side and the receiving side in each game to construct vectors. A feature vector was formed by subtracting the vector of the receiving side from that of the serving side. Furthermore, we defined the labels based on the outcome of each game: if the serving side won the game, the label was set to 1; if the receiving side won, the label was set to 0.Article
CAS
PubMed
Google Scholar - Wanli OuyangIn this study, we collected data from international badminton competitions from 2019 to 2023, aiming to statistically analyze the frequency of each technical action in each rally of the competitions. The considered technical actions include High, Smash, Dribble, etc., totaling 23, see Methods for details. The frequency of these technical actions was used as feature vectors to analyze the winning patterns of both the serving and receiving sides, further aiming to build a model capable of predicting competition outcomes.
- This study initiates from the perspective of the serving and receiving players’ technical actions in each game, elucidating the respective key technical action frequency factors of both parties in the competition process and constructing a predictive model based on these insights for subsequent researchers and coaches to study and reference. By meticulously analyzing the frequency of 23 distinct technical actions in international badminton competitions and employing a sophisticated Random Forest algorithm, we have not only identified the pivotal technical actions that significantly impact match outcomes but have also quantified their influence through the calculation of SHAP values. This innovative approach has allowed us to reveal that technical actions such as ‘Net Front’, ‘Slice/Drop’, and ‘Push’ play a crucial role in determining the victory, offering a nuanced understanding that transcends traditional analyses. The competition prediction models were also constructed measured by Sensitivity, Specificity, Accuracy, PPV and NPV, which could be directed applied by future related researchers and athletes. In this study, through precise data analysis and the construction of machine learning models, we unveiled the significant impact of three technical actions—‘Net Front’, ‘Slice/Drop’, and ‘Push’—on predicting the outcomes of badminton matches. These actions demonstrated similar importance across models analyzing both male and female players’ data, ranking them among the top five key factors influencing match results. This finding not only deepens our understanding of the tactical nuances in badminton but also provides invaluable guidance for coaches and athletes in formulating targeted training and competition strategies. Furthermore, our results align closely with findings in the existing literature, which commonly acknowledges the critical role of ‘Net Front’ techniques for short net play, ‘Slice/Drop’ for executing drop shots, and ‘Push’ for driving shots in winning badminton matches27,28. Specifically, ‘Net Front’ plays a decisive role in controlling the pace of the game and forcing opponents to make mistakes, while ‘Slice/Drop’ and ‘Push’ are primarily utilized to disrupt the opponent’s defensive setup and create offensive opportunities. This concurrence further validates the effectiveness of our study’s outcomes, demonstrating the potential of data-driven approaches in revealing the impact of core technical actions in high-level badminton competitions.We implemented a random forest classifier using the scikit-learn package in Python 3.97 programming language (https://www.python.org/). To generate the training (70%) and test (30%) datasets, we split the dataset using the train_test_split function, which randomly partitions a dataset into training and test subsets with test_size = 0.3 parameter. We applied this function to each sex individually. To identify the key technical actions critical for predicting match outcomes, we first constructed a random forest classifier using the default parameters based on the entire training dataset18. We extracted the feature importance parameters from this classifier and sorted the features according to their importance. Subsequently, the features were incrementally included in the default random forest classifier based on their ranked importance. This process was validated using a 5-fold cross-validation method19 to determine the optimal number of technical actions for describing the model features. To build a random forest classifier with the best hyperparameters, we implemented the exhaustive grid search approach using the GridSearchCV function to the training dataset with five-fold cross-validation. A total of 10,000 random forest classifier models were evaluated with different combinations of hyperparameters: max_features=”auto”; n_estimators ranging from 100 to 1,000 with an interval of 100; max_depth ranging from 2 to 20 with an interval of 2; min_samples_leaf ranging from 2 to 20 with an interval of 2; and min_samples_split ranging from 2 to 20 with an interval of 2. As a result, we selected the male model with n_estimators = 600, max_depth = 8, min_samples_leaf = 2 and min_samples_split = 2 hyperparameters, which showed the highest average AUC at 0.9656. Also, the female model was built with n_estimators = 1000, max_depth = 12, min_samples_leaf = 2 and min_samples_split = 8 hyperparameters, which showed the highest average AUC at 0.8950. After identifying the optimal hyperparameters, the SHAP (Shapley Additive Explanations) values are used to evaluate the contribution and direction of features20,21. Finally, the indices of sensitivity, specificity, accuracy, positive predictive value (PPV) and negative predictive value (NPV) were utilized to evaluate the performance of finalized models22,23.
- Gomez, M., Rivas, F., Connor, J. D. & Leicht, A. S. performance differences of temporal parameters and point outcome between elite men’s and women’s badminton players according to match-related contexts. Int. J. Environ. Res. Public Health 16(21), (2019).
- School of Physical Education and Sport Training, Shanghai University of Sport, Shanghai, China
- Yi ShengSheng, Y., Yi, Q., Gómez-Ruano, M. & Chen, P. The influence of technical and contextual variables of the last stroke on point outcome in men’s and women’s singles badminton. Front. Psychol. 12, 802179 (2021).
- Putra, V. G. V. & Irwan, Mohamad, J. N. A novel mathematical model of the badminton smash: Simulation and modeling in biomechanics. Comput. Methods Biomech. BioMed. Eng. 27(4), 538–545 (2024).Recent research has highlighted the crucial role of mutual counteraction between distinct tactical styles in determining match outcomes8. Players employing specific tactical styles may inherently possess advantages or disadvantages against opponents with contrasting styles5. Understanding this dynamic is pivotal for devising effective strategies. The frequency of technical actions has emerged as a critical indicator of tactical approaches, shedding light on the complexities of gaining a strategic edge in badminton9.
- In this study, we focus on the top ten male and female singles players as ranked by the Badminton World Federation (BWF), selecting videos of their matches from Super750-level and higher competitions between 2019 and 2023 as observational data. A total of 303 matches were analyzed, including 153 men’s singles matches encompassing 358 games, and 150 women’s singles matches encompassing 344 games. The selected matches cover major international badminton tournaments, such as the 2020 Tokyo Olympics, the World Championships, the All England Open, the Sudirman Cup, the Thomas Cup, and the Uber Cup. The match videos were primarily sourced from national team archives, Bilibili, CCTV broadcasts, and he official website of the Badminton World Federation (BWF).Wang, C. Optimization of sports effect evaluation technology from random forest algorithm and elastic network algorithm. PloS One. 18(10), e0292557 (2023).
- Website availability: https://dachengzi.shinyapps.io/prediction_model_on_babminton/.Buergel, T. et al. Metabolomic profiles predict individual multidisease outcomes. Nat. Med. 28(11), 2309–2320 (2022).
- Sorry, a shareable link is not currently available for this article.McHugh, M. L. Interrater reliability: The kappa statistic. Biochemia Med. 22 (3), 276–282 (2012).
- This study successfully developed well-performing badminton match outcome prediction models based on the interplay and counteraction among different tactical styles, and through a user-oriented web application, achieved visualization and interaction of the model, highlighting the significant impact of key technical actions—Net Front, Slice/Drop, and Push—on match outcomes and providing a scientific basis for badminton training and match strategies.Article
Google Scholar - Chowell, D. et al. Improved prediction of immune checkpoint blockade efficacy across multiple cancer types. Nat. Biotechnol. 40(4), 499–506 (2022).PubMed Google Scholar
DOI: https://doi.org/10.1038/s41598-025-87610-7
Author information
Authors and Affiliations
Cheng Liu, Ru Wang & Peijie Chen