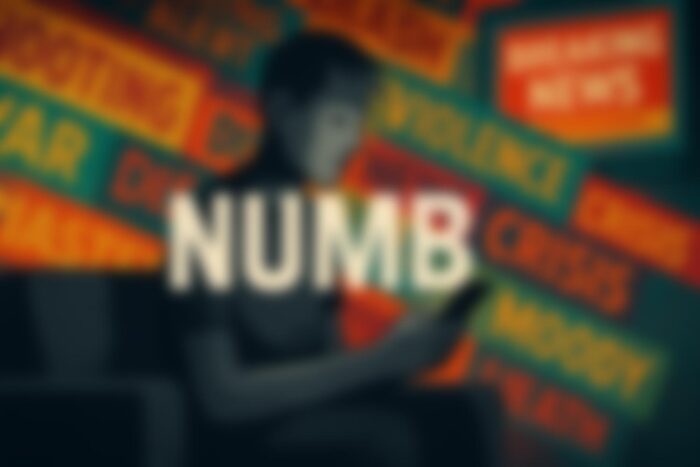
During a speech at a Turning Point USA event over the weekend, conservative political pundit Tucker Carlson spoke dismissively about the issue of transgender athletes – biological men – competing in women’s sports in light of bigger problems facing the country.
The breadth of Carlson’s commentary is that while men competing in women’s sports is wrong, it’s not an important issue. In his estimation, Republicans and conservatives are exaggerating their victories on the matter and losing focus on bigger problems in the United States.
“You spend all day telling me that it’s so important that boys not play on girls’ soccer teams or whatever. I agree. I hate the tranny stuff,” Carlson said. “But, I don’t know, it kind of feels like you’re feeding me appetizers. At some point, I want to look around and see a better country.”
Political pundit Tucker Carlson (left) gave a speech during a Turning Point USA event where he dismissed transgender athletes (like Lia Thomas, right) competing in women’s sports as unimportant.
(Imagn Images)
After a quick aside to suggest that the United States should employ the military to stop drugs from coming into the country, he circled back to the transgender issue.
“Why are my cities disgusting? I don’t even want to go there. It smells like weed and halal food. And you’re lecturing me about how it’s a great moral victory that I kicked the boys off the girls’ soccer team,” Carlson said. “Good. I’m so glad. But let’s do the real stuff like making New York [City] livable.”
Here’s Carlson’s full rant:
I understand where Carlson is coming from. I really do. At the end of the day, boys and men in girls’ and women’s sports don’t affect as many Americans as, say, the opiod crisis or rampant homelessness. Anyone who has spent time in the biggest cities in the country – New York and Los Angeles – understands this.
Transgender Athletes Are A Symptom Of A Larger Issue
The transgender athlete debate – which is an adjunct of the Democrats’ insistence on unwavering support for gender ideology – represents a far deeper cultural issue.
The essential platform of the modern Democratic Party in America boils down to a simple solution for everything: compassion. Democrats and their left-wing allies believe that compassion should trump everything else when it comes to policy.
But Democrats don’t actually understand the word compassion.
They believe that the compassionate response to homeless people – nearly all of whom are helplessly addicted to drugs or suffering from intense mental illness – is to let them continue to do drugs and sleep on the streets. In their minds, it’s cruel to put them in jail or force them into shelters or rehab facilities.
Democratic policies on transgender athletes in women’s sports highlight their other terrible policy positions, which have led to a homelessness crisis across the United States.
(Allen J. Schaben/Los Angeles Times via Getty Images)
They apply the same logic to the transgender issue. A young boy believes he’s actually a girl? OK, let him be a girl. That’s what Democrats think. The compassionate thing to do is to indulge that delusion and bend over backwards to make the rest of society indulge the delusion as well. Make no mistake: a boy or a girl believing they are living in the wrong body is a delusion in the vast majority of cases. It needs to be treated as such.
Democrats are, essentially, bad parents.
Compassion Is Bad Parenting
Think about it this way. You’re a parent. Your child is screaming because he or she wants to eat ice cream for dinner and isn’t interested in the broccoli on the plate. What is the compassionate thing to do in this situation? Well, according to Democrats, give the kid ice cream. That’s what they want, so give it to them.
Does anyone believe that’s actually the correct parenting move? Of course not. The real compassion is tough love. You want to eat ice cream for dinner? Too bad. It’s not good for you, and you’ll thank me later.
Now, you might be thinking that this analogy doesn’t work for these complex situations. But I disagree. In fact, I think it’s the perfect analogy, especially because that’s how the Democratic Party views itself: They believe it’s the role of the government to serve as the parents of children. Look at their policies if you think I’m wrong. Democrats, at their core, believe that citizens have no control over their own lives, like children. Republicans, on the other hand, generally preach personal accountability, like adults.
But what does any of this have to do with Tucker’s rant? The problem isn’t just that some males compete in women’s sports. Yes, it’s terrible that women are forced to share locker rooms and bathrooms with men in the name of gender ideology. Or have to sacrifice their athletic opportunities. But it’s the root of why that’s happening that underscores the insidiousness of the issue.
Republicans focusing on this issue, and having the overwhelming majority of Americans agree with them, is an extremely important cultural victory that has already started to change the narrative.
Many Americans look the other way while Democrat-run cities fall into complete disarray thanks to drugs, mental illness, homelessness and general mismanagement. But when Democrats say that boys can be girls and that they can use whatever bathroom they choose and compete against whomever they want, Americans are standing up and saying, “Enough!”
Adults Acting Like Adults
This is about much more than just keeping men out of women’s sports. It’s a signal that the adults in the room are finally starting to act like adults. The days of letting your kid eat ice cream, play video games and contribute nothing are over. It’s time to teach them personal responsibility and, sometimes, that means being the bad guy – at least at the moment.
Because long-term, tough love early in life leads to an easier time later in life. It’s a simple concept, one that’s been known for hundreds – if not thousands – of years, but one that we seem to have forgotten in these modern times. The win of keeping men out of women’s sports is not a small victory, as Carlson suggests. It’s a major victory that signals a broader cultural shift that America very much needed.
People are, hopefully, waking up to the disastrous policy decisions made by Democrats. Without winning the battle on transgender athletes, Republicans couldn’t have exposed the other problems created by Democrats. American citizens are looking at Democrat politicians who are still pushing gender ideology, and supporting men in women’s sports, and saying, “If you believe something that ridiculous, how can I trust you on other important issues?”
That’s why Tucker Carlson missed the mark. He downplays the importance of the transgender athlete issue because there are, in his mind, more important issues facing the country. He’s right about that, but also wrong. Winning the battle against transgender athletes in women’s sports and gender ideology sets up Republicans to start gaining important ground in the culture war. You don’t have to win every battle to win a war. But you have to win the important ones.
This is one of those very important victories.
0