Abstract National Science Centre in Poland supported preparing this article with a research grant (UMO-2020/39/B/HS6/00685) and a scholarship awarded to M.B. by the Foundation for Polish Science (FNP). The funder had no role in study design, data collection, analysis, publishing decisions, or manuscript preparation. The project was conducted in AMU Psychophysiology Lab: Positive Gaming & […]
Abstract
Background & Summary
PubMed
Google Scholar
MAPI Research Institute. Generalized Anxiety Disorder – 7 (GAD-7). Available at: https://eprovide.mapi-trust.org/instruments/generalized-anxiety-disorder-7 (accessed July 2023).Dweck, C. S. Mindset: The New Psychology of Success. Random House (2006).
-
1)
Crum, A. J., Salovey, P. & Achor, S. Rethinking stress: the role of mindsets in determining the stress response. J. Pers. Soc. Psychol. 104, 716 (2013).
- 2)
- 3)
-
4)
Bailey, H. Open Broadcasting Software. Retrieved from https://obsproject.com/ (2018).
Received:
Methods
MATH
Google Scholar
Participants
De France, K. & Hollenstein, T. Assessing emotion regulation repertoires: The regulation of emotion systems survey. Pers. Individ. Differ. 119, 204–215 (2017).
Ethics Information
The physiological and behavioral data, in a CSV format, constitute 366 GB of space32. The files are grouped into eight subcomponents with a maximum size of 50 GB due to OSF storage restrictions. The component’s name indicates for which study stage (S1 vs S3) and participants and which person they refer to. For instance, the name ‘Physio_S3_225_300’ indicates that the component included psychophysiological and behavioral data from Stage 3 for participants from 225 to 300. The component contains a set of CSV files for particular subjects. All psychophysiological and behavioral signals recorded during the experiment for each individual are available in a single CSV datafile named “S < stage_id > _P < participant_id >,” where “S” stands for study stage, “P” for participants, e.g., S1_P10.csv, or S3_P224.csv. The “< particpant_id >” is a natural number identifying a participant.
Procedure
Article
PubMed
PubMed Central
Google Scholar
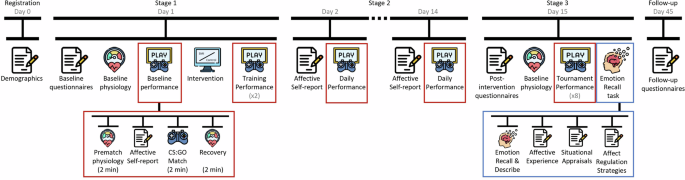
Stage 1
Internal/external validity: our study offered a unique blend of internal and external validity through the use of controlled experiments paired with real-world outcomes. It included a thorough evaluation of affective and physiological dynamics and implemented a robust, theory-driven intervention.
Zhang, Z. et al. Multimodal spontaneous emotion corpus for human behavior analysis. In IEEE Conf. Comput. Vision Pattern Recognit. 3438–3446 (2016).Article
PubMed
Google Scholar
Stage 2
Article
MATH
Google Scholar
Article
PubMed
Google Scholar
Stage 3
Epel, E. S. et al. More than a feeling: A unified view of stress measurement for population science. Front. Neuroendocrinol. 49, 146–169 (2018).Kroenke, K., Spitzer, R. L. & Williams, J. B. The Patient Health Questionnaire-2: validity of a two-item depression screener. Med. Care 41, 1284–1292 (2003).Some information about our study is detailed in the published registered report6 – which presents hypothesis testing related to the effects of the Synergistic Mindsets Intervention – including a comprehensive description of the sampling procedures, study procedure, questionnaires, and physiological data. In this paper, we provide additional details on open-text responses, video recordings, and behavioral data. Furthermore, we include new information regarding data quality and present how the measures changed over the course of laboratory visits. Finally, to enhance the usability of the CEPAV dataset, we standardized and merged the physiological and behavioral data collected from three different devices (each with distinct data formats and sampling rates) and uploaded the resulting user-friendly files instead of the raw data.
Measures
Questionnaires
Article
PubMed
MATH
Google Scholar
Open-Text answers
You can also search for this author in
PubMed Google Scholar
Other self-reports
Demographics
Article
CAS
PubMed
PubMed Central
MATH
Google Scholar
Performance
Brytek-Matera, A. & Kozieł, A. The body self-awareness among women practicing fitness: a preliminary study. Pol. Psychol. Bull. 46, 104–111 (2015).
Video data
Video recordings
Crum, A. J., Akinola, M., Martin, A. & Fath, S. The role of stress mindset in shaping cognitive, emotional, and physiological responses to challenging and threatening stress. Anxiety Stress Coping 30, 379–395 (2017).
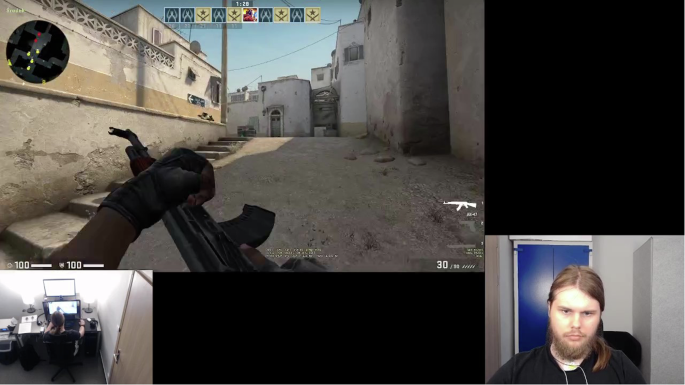
Gameplay recordings
Article
CAS
PubMed
PubMed Central
Google Scholar
Physiological measures
Impedance cardiography and electrocardiography
Article
CAS
PubMed
MATH
Google Scholar
Blood pressure
Shui, X. et al. A dataset of daily ambulatory psychological and physiological recording for emotion research. Sci. Data 8, 161 (2021).
Behavioral measures
Kroenke, K., Spitzer, R. L., Williams, J. B., Monahan, P. O. & Löwe, B. Anxiety disorders in primary care: prevalence, impairment, comorbidity, and detection. Ann. Intern. Med. 146, 317–325 (2007).
Data preprocessing
Koelstra, S. et al. Deap: A database for emotion analysis; using physiological signals. IEEE Trans. Affective Comput. 3, 18–31 (2011).Histograms Presenting Ranges of Means of Collected Signals. Panel A presents data from Stage 1; Panel presents data from Stage 3. HR – Heart rate, bpm, SBP – Systolic Pressure, mmHg; DBP – Diastolic Pressure mmHg; SV – Stroke Volume, ml; LVET – Left Ventricular Ejection Time, ms; PI- Pulse Interval, ms; MS – Maximum Slope; mmHg/s; CO – Cardiac Output; l/min; TPR – Total Peripheral Resistance Medical Unit, mmHg.min/l; TPRCGS – Total Peripheral Resistance CGS; dyn.s/cm5; wr – right wrist movement, custom units; tl – left thigh movement, custom units; tr – right thigh movement, custom units.Article
PubMed
PubMed Central
MATH
Google Scholar
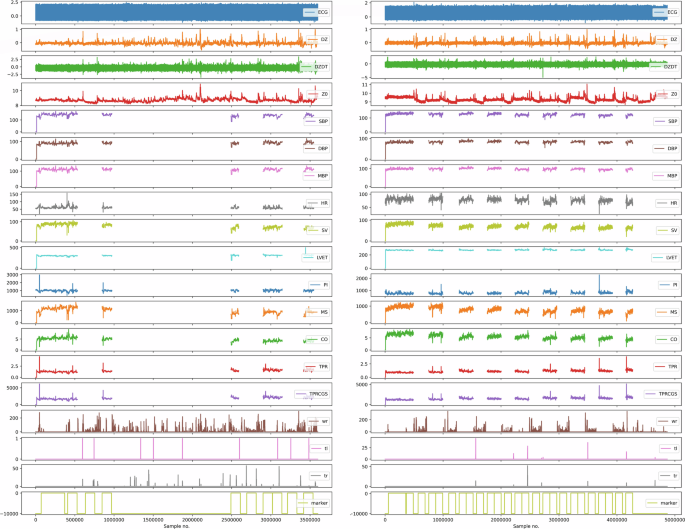
PubMed Google Scholar
Maciej Behnke, Wadim Krzyżaniak, Patrycja Chwiłkowska, Szymon Jęśko Białek, Maciej Kłoskowski, Patryk Maciejewski & Kacper Szymański
Data Records
Dataset Structure
Self-reports and metadata
Ab. Aziz, N. A. K. T. et al. Asian affective and emotional state (A2ES) dataset of ECG and PPG for affective computing research. Algorithms 16, 130 (2023).
Video data
Melhart, D., Liapis, A. & Yannakakis, G. N. The arousal video game annotation (AGAIN) dataset. IEEE Trans. Affective Comput. 13, 2171–2184 (2022).
Physiological and behavioral data
Jankowski, K. S. Is the shift in chronotype associated with an alteration in well-being? Biol. Rhythm Res. 46, 237–248 (2015).
Single physiological file structure
Technical Validation
Missing data
Questionnaires reliability
Koldijk, S., Sappelli, M., Verberne, S., Neerincx, M. A. & Kraaij, W. The swell knowledge work dataset for stress and user modeling research. In Multimodal Interaction (2014).
Physiological data – qualitative validation
Physiological data – quantitative validation
Article
MATH
Google Scholar
Yeager, D. S. et al. A synergistic mindsets intervention protects adolescents from stress. Nature 607, 512–520 (2022).Li, J. Psychometric properties of Ten-Item Personality Inventory in China. Chin. J. Health Psychol. 21, 1688–1692 (2013).
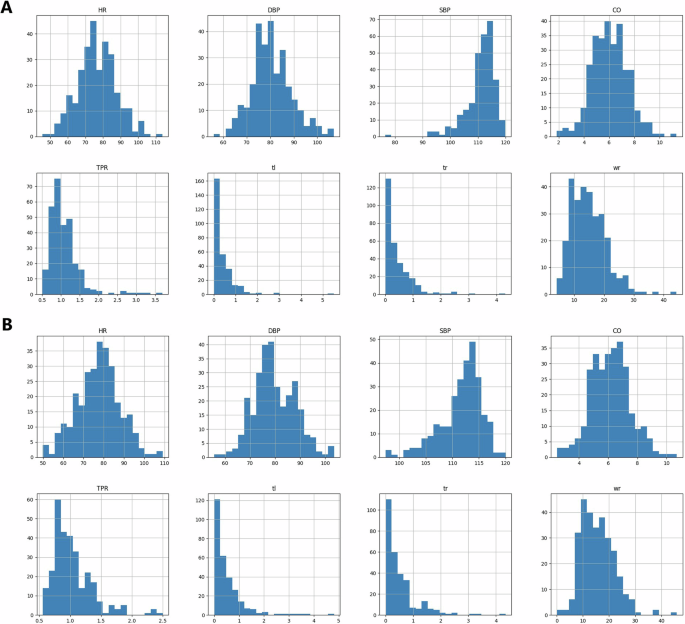
Summary of previously completed analyses
Summary of the physiological, affective, and behavioral activity during the competitive esports
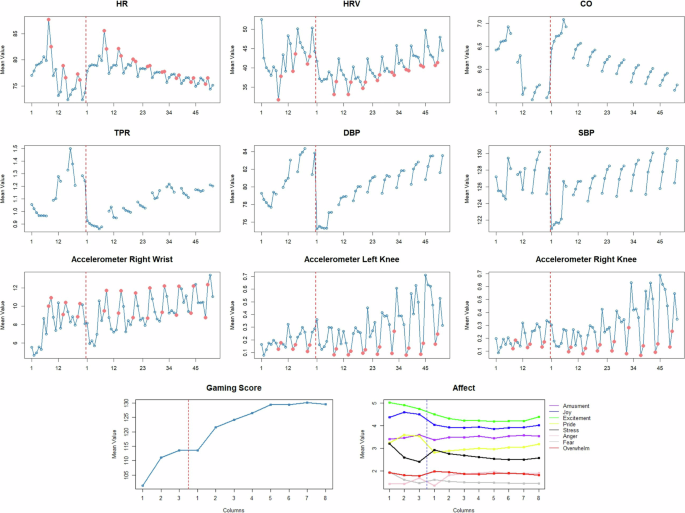
Usage Notes
PubMed
Google Scholar
Declaration of Generative AI and AI-assisted technologies in the writing process
Christy, A. G., Schlegel, R. J. & Cimpian, A. Why do people believe in a “true self”? The role of essentialist reasoning about personal identity and the self. J. Pers. Soc. Psychol. 117, 386–416 (2019).
References
- Article
PubMed
Google Scholar - Article
PubMed
Google Scholar - Department of Psychology, Stanford University, Stanford, USAGosling, S. D., Rentfrow, P. J. & Swann, W. B. Jr. A very brief measure of the Big-Five personality domains. J. Res. Pers. 37, 504–528 (2003).
- Robins, R. W., Hendin, H. M. & Trzesniewski, K. H. Measuring global self-esteem: Construct validation of a single-item measure and the Rosenberg Self-Esteem Scale. Pers. Soc. Psychol. Bull. 27, 151–161 (2001).Moore, L. J., Vine, S. J., Wilson, M. R. & Freeman, P. The effect of challenge and threat states on performance: An examination of potential mechanisms. Psychophysiology 49, 1417–1425 (2012).
- Kroenke, K., Spitzer, R. L. & Williams, J. B. W. The PHQ-9: Validity of a brief depression severity measure. J. Gen. Intern. Med. 16, 606–613 (2001).Markova, V., Ganchev, T. & Kalinkov, K. Clas: A database for cognitive load, affect, and stress recognition. In Biomed. Innov. Appl. (2019).
- Article
Google Scholar
Other psychophysiological datasets related to affective manipulations exist (see Tables 1–3), but these datasets are usually related to one of the data types included in CEPAV. We reviewed existing openly available datasets of physiological responses to affective manipulations. Compared to the CEPAV strengths, we found that only four databases included physiological, behavioral, and video data along with individual differences measures8,9,10,11. Seven databases included data collected on multiple occasions10,12,13,14,15,16,17. Only one database included more participants than the CEPAV18 dataset. Finally, we found five datasets that included gaming, including simple labyrinth games9, racing games19,20 shooter games platform games19, FIFA21, and League of Legends16. Only one dataset included data on participants’ performance16, while other datasets used gaming as a situational context for the study. - Crucianelli, L., Enmalm, A. & Ehrsson, H. H. Interoception as independent cardiac, thermosensory, nociceptive, and affective touch perceptual submodalities. Biol. Psychol. 172, 108355 (2022).Hsu, Y. L., Wang, J. S., Chiang, W. C. & Hung, C. H. Automatic ECG-based emotion recognition in music listening. IEEE Trans. Affective Comput. 11, 85–99 (2017).
- De France, K. & Hollenstein, T. Emotion regulation and relations to well-being across the lifespan. Dev. Psychol. 55, 1768–1778 (2019).
- The upper-body recordings in an MP4 format, full HD resolution (1920 × 1080) constitute 820 GB of space31. The files are grouped into 19 components with a maximum size of 50 GB due to OSF storage restrictions. The component’s name indicates for which study stage (S1 vs S3) and participants and which person they refer to. For instance, the name ‘Video_S3_255_280’ indicates that the component included a video record from Stage 3 for participants from 255 to 280. To ease the video data analysis, we included the time intervals for each experimental epoch in the “CEPAV_data/video_timing” sheet24 (e.g., for Stage 1 for Participant 8, the baseline starts at 1:17 minutes of video).Article
CAS
PubMed
PubMed Central
Google Scholar - Hughes, B. M., Lü, W. & Howard, S. Cardiovascular stress-response adaptation: Conceptual basis, empirical findings, and implications for disease processes. Int. J. Psychophysiol. 131, 4–12 (2018).The CEPAV dataset is publicly available at the Open Science Framework (OSF) repository1.
- Article
PubMed
Google Scholar
You can also search for this author in
PubMed Google Scholar - Subramanian, R. et al. ASCERTAIN: Emotion and personality recognition using commercial sensors. IEEE Trans. Affective Comput. 9, 147–160 (2016).We evaluated the quality of the signal with the Signal to Noise Ratio (SNR). In order to calculate SNR across the diverse physiological signals, we used an algorithm based on the autocorrelation function of the signal, using the second-order polynomial for fitting the autocorrelation function curve34. We used this approach in our previous project18. The script we used for calculating SNR is available in the Code Component30 and project’s GitHub repository (https://github.com/psychosensing/CEPAV). The SNR coefficients for all channels for Stages 1 and 3 are available in the “CEPAV_data/SNR” sheet24.
- Article
MATH
Google Scholar
Soleymani, M., Lichtenauer, J., Pun, T. & Pantic, M. A multimodal database for affect recognition and implicit tagging. IEEE Trans. Affective Comput. 3, 42–55 (2011). - Abbey, J. D. & Meloy, M. G. Attention bydesign: Using attention checks to detect inattentive respondentsand improve data quality. J. Oper. Manag. 53, 63–70 (2017).
- Article
CAS
PubMed
PubMed Central
Google Scholar
Article
PubMed
Google Scholar - Article
CAS
PubMed
PubMed Central
Google Scholar - Schleider, J. L., Mullarkey, M. C. & Weisz, J. R. Virtual reality and web-based growth mindset interventions for adolescent depression: Protocol for a three-arm randomized trial. JMIR Res. Protoc. 8, e13368 (2019).Wylie, M. S. et al. Momentary emotion regulation strategy use and success: Testing the influences of emotion intensity and habitual strategy use. Emotion 22, 83–95 (2022).
- Given the expense associated with assembling comprehensive multimodal datasets, there is growing interest in reusing existing datasets, a practice that remains underutilized due to the scarcity of openly shared raw data. For data reuse, it is particularly important to share the raw data rather than the pre-processed data used for analysis, as raw data are needed in order to fully replicate analyses and harness the full potential of the data. This is particularly critical in fields like affective computing, where developing algorithms capable of understanding and adapting to human emotions relies on access to diverse and detailed descriptors of emotional responses. Such openness and transparency not only enhance the potential for interdisciplinary collaboration but also significantly enrich the resources available for affective science. By providing raw, unprocessed data, researchers enable more nuanced analysis, fostering advancements in understanding the complexities of affective phenomena.Ware, J. E. Jr. & Sherbourne, C. D. The MOS 36-item short-form health survey (SF-36): I. Conceptual framework and item selection. Med. Care 30, 473–483 (1992).
- You can also search for this author in
PubMed Google ScholarCorrespondence to
Maciej Behnke. - Behnke, M. et al. CEPAV Dataset, Raw_Physio Component. Open Science Framework https://doi.org/10.17605/OSF.IO/HKDUY (2024).Physiological and behavioral data were exported from the acquisition formats by the first author (MB). We used different acquisition software; therefore, the exported data had to be integrated into a common format. The exported TXT and CSV files were preprocessed using Python28,29 scientific libraries (e.g., pandas 2.2.2, numpy 1.26.4; see Code Availability, for detailed information). In addition to scripts for processing the typical data, we also added scripts for handling problematic cases and exceptions.
- In our initial publication, we tested the effects of the Synergistic Mindsets Intervention (SMI) compared to a control intervention6. The SMI was positively received, leading participants to adopt more advantageous stress mindsets, more favourable appraisals of the esports tournament, and an increased application of reappraisal strategies for emotion regulation. Despite these positive outcomes, the high-stakes nature of the esports competition was perceived as an enjoyable challenge rather than a negative stressor, reducing the potential for the SMI to significantly influence affective and physiological reactions. The absence of a negative physiological stress response meant there was very little for the intervention to modulate. Consequently, no significant changes were noted in affective responses or gaming performance due to the intervention. Access to the research code, dataset, and findings can be found elsewhere6.
- We converted the raw acquired data (obtained with proprietary acquisition software) into a consistent format and saved it in CSV files. All signals were resampled to 1 kHz, using the previous neighbor interpolation method. Signals from different devices were time-synchronized using synchronization markers generated by VU-AMS and Finometer devices during experiments. We marked the baselines, matches, and recoveries within the files. Finally, data across studies were exported to normalized form, consisting of a header, predefined file structure, and standardized subject naming convention. The description of labels used for tagging specific epochs is available in the “CEPAV_data” file24, the “epoch_name” sheet.
- You can also search for this author in
PubMed Google Scholar - Roksa, J. & Kinsley, P. The role of family support in facilitating academic success of low-income students. Res. High. Educ. 60, 415–436 (2019).
- Esports refers to competitive video gaming where individuals compete against each other in organized tournaments for prize money. Here, we present the Competitive Esports Physiological, Affective, and Video (CEPAV) dataset, in which 300 male Counter Strike: Global Offensive gamers participated in a study aimed at optimizing affect during esports tournament1. The CEPAV dataset includes (1) physiological data, capturing the player’s cardiovascular responses from before, during, and after over 3000 CS: GO matches; (2) self-reported affective data, detailing the affective states experienced before gameplay; and (3) video data, providing a visual record of 552 in-laboratory gaming sessions. We also collected (affect-related) individual differences measures (e.g., well-being, ill-being) across six weeks in three waves. The self-reported affective data also includes gamers’ natural language descriptions of gaming affective situations. The CEPAV dataset provides a comprehensive resource for researchers and analysts seeking to understand the complex interplay of physiological, affective, and behavioral factors in esports and other performance contexts.
- Saganowski, S. et al. Emognition dataset: Emotion recognition with self-reports, facial expressions, and physiology using wearables. Sci. Data 9, 158 (2022).Moore, L. J., Vine, S. J., Wilson, M. R. & Freeman, P. Reappraising threat: How to optimize performance under pressure. J. Sport Exerc. Psychol. 37, 339–343 (2015).
- MAPI Research Institute. Patient Health Questionnaire (PHQ). Available at: https://eprovide.mapi-trust.org/instruments/patient-health-questionnaire (accessed July 2023).van Lien, R., Neijts, M., Willemsen, G. & de Geus, E. J. Ambulatory measurement of the ECG T‐wave amplitude. Psychophysiology 52, 225–237 (2015).
- Wang, Z. et al. Reliability and validity of the Chinese version of Beck Depression Inventory-II among depression patients. Chin. Ment. Health J. 25, 476–480 (2011).
- MATH
Google Scholar
Visualization Single Physiological File Structure for Stage 1 (left panel) and 3 (right panel). ECG- electrocardiogram, mV; Z0 – Average thorax impedance, ohm; dZ – Change in impedance due to respiration and heartbeat, ohm; dZ/dt – Impedance CardioGram, ohm/s; SBP – Systolic Pressure, mmHg; DBP – Diastolic Pressure, mmHg; MAP- Mean Pressure, mmHg; HR – Heart rate, bpm; SV – Stroke Volume, ml; LVET – Left Ventricular Ejection Time, ms; PI- Pulse Interval, ms; MS – Maximum Slope; mmHg/s; CO – Cardiac Output; l/min; TPR – Total Peripheral Resistance Medical Unit, mmHg.min/l; TPRCGS – Total Peripheral Resistance CGS; dyn.s/cm5; wr – right wrist movement, custom units; tl – left thigh movement, custom units; tr – right thigh movement, custom units. - Ringeval, F., Sonderegger, A., Sauer, J. & Lalanne, D. Introducing the RECOLA multimodal corpus of remote collaborative and affective interactions. In Face Gesture Recognit. (2013).
- Article
PubMed
PubMed Central
MATH
Google Scholar - Meade, A. W. & Craig, S. B. Identifying carelessresponses in survey data. Psychol. Methods 17, 437–455 (2012).
- Article
CAS
PubMed
MATH
Google Scholar
Shifts in Mean Levels of Affective Measures. Red line separates Stage 1 and Stage 3 laboratory visits. HR – Heart rate, bpm, SBP – Systolic Pressure, mmHg; DBP – Diastolic Pressure mmHg; CO – Cardiac Output; l/min; TPR – Total Peripheral Resistance Medical Unit, mmHg.min/l. - O’Brien, S. T. et al. SEMA3: A free smartphone platform for daily life surveys. Behav. Res. Methods 1–16 (2024).Carstensen, L. L., Shavit, Y. Z. & Barnes, J. T. Age advantages in emotional experience persist even under threat from the COVID-19 pandemic. Psychol. Sci. 31, 1374–1385 (2020).
- Article
Google Scholar
Beili, Z. Introduction to the POMS scale and the short-form Chinese norm. J. Tianjin Sports Inst. 35–37 (1995). - Article
PubMed
Google Scholar
Article
Google Scholar - Raheel, A., Majid, M. & Anwar, S. M. DEAR-MULSEMEDIA: Dataset for emotion analysis and recognition in response to multiple sensorial media. Inf. Fusion 65, 37–49 (2021).
- Article
MATH
Google Scholar
Article
Google Scholar - During the preparation of this work, the authors used Grammarly and ChatGPT4.0 to improve the readability and language. After using this tool/service, the authors reviewed and edited the content as needed and take full responsibility for the publication’s content.Costa, P. & McCrae, R. Revised NEO Personality Inventory (NEO-PI-R) and NEO Five Factor Inventory (NEO-FFI). Professional manual. Psychological Assessment Resources (1992).
- Article
PubMed
MATH
Google Scholar
Moore, L. J., Wilson, M. R., Vine, S. J., Coussens, A. H. & Freeman, P. Champ or chump?: Challenge and threat states during pressurized competition. J. Sport Exerc. Psychol. 35, 551–562 (2013). - DOI: https://doi.org/10.1038/s41597-024-04364-z
- Behnke, M. et al. CEPAV Dataset, Videos Component. Open Science Framework https://doi.org/10.17605/OSF.IO/QKD5B (2024).
- Article
PubMed
MATH
Google Scholar
Fredrickson, B. L. Positive emotions broaden and build. Adv. Exp. Soc. Psychol. 47, 1–53 (2013). - You can also search for this author in
PubMed Google ScholarCudo, A., Montag, C. & Pontes, H. M. Psychometric assessment and gender invariance of the Polish version of the Gaming Disorder Test. Int. J. Ment. Health Addict. 1–24 (2022). - Schleider, J. L. et al. Acceptability and utility of an open-access, online single-session intervention platform for adolescent mental health. JMIR Ment. Health 7, e20513 (2020).
- Smerdov, A. et al. Collection and validation of psychophysiological data from professional and amateur players: A multimodal esports dataset. arXiv preprint arXiv:2011.00958 (2020).
- Kutt, K. et al. BIRAFFE2: A multimodal dataset for emotion-based personalization in rich affective game environments. Sci. Data 9, 274 (2022).Open Access This article is licensed under a Creative Commons Attribution 4.0 International License, which permits use, sharing, adaptation, distribution and reproduction in any medium or format, as long as you give appropriate credit to the original author(s) and the source, provide a link to the Creative Commons licence, and indicate if changes were made. The images or other third party material in this article are included in the article’s Creative Commons licence, unless indicated otherwise in a credit line to the material. If material is not included in the article’s Creative Commons licence and your intended use is not permitted by statutory regulation or exceeds the permitted use, you will need to obtain permission directly from the copyright holder. To view a copy of this licence, visit http://creativecommons.org/licenses/by/4.0/.
- Article
PubMed
MATH
Google Scholar
For open-text responses, we initially reviewed and corrected any typographical and spelling mistakes. Subsequently, we translated these responses into English using DeepL Translator (DeepL GmbH, Cologne, Germany). Two judges (KS, MB, MK, or SJB) then compared the English translations to the original Polish texts, making adjustments for any clear translation errors. Three judges deliberated on more complex issues and resolved them through consensus. - Article
CAS
PubMed
PubMed Central
MATH
Google Scholar
You can also search for this author in
PubMed Google Scholar - Article
PubMed
PubMed Central
Google Scholar
Thong, J. T. L., Sim, K. S. & Phang, J. C. H. Single‐image signal‐to‐noise ratio estimation. Scanning 23, 328–336 (2001). - Zamariola, G. et al. Relationship between interoceptive accuracy, interoceptive sensibility, and alexithymia. Pers. Individ. Differ. 125, 14–20 (2018).You can also search for this author in
PubMed Google Scholar - We collected the number of kills, kills’ assists, and deaths and the match scores as determined by the Counter-Strike: Global Offensive scoring system, which factors in the difficulty of the weapon used and the points earned for each enemy bot eliminated. A higher score reflects superior performance. Gamers’ tournament performance was primarily evaluated based on their total score, making it the main performance index. However, other metrics—kills, assists, and deaths—can provide insight into the participant’s strategy. For instance, a high total score paired with a high number of deaths may indicate a risk-taking approach. Analyzing which strategies proved optimal or suited individual gamers could be valuable for esports coaches, helping them tailor training and game plans effectively. In Stage 2, participants were asked to log their daily match scores, simulating the conditions of the upcoming tournament.Download references
- However, the dataset comes with certain limitations. First, this dataset cannot be employed to investigate differences between sexes, ethnicities, or between the group ages, as all participants were male Caucasian young adults. Second, our investigation was confined to affective reactions within a single esports (Counter Strike: Global Offensive) context. Third, as noted in the missing data section, the dataset lacks some data due to technical constraints (e.g., ICG missing due to electrode detachment), lack of consent to share data, and human errors (e.g., not starting data collection for accelerometers). Lastly, the dataset represents a secondary use of data initially collected for a previously published independent study.Accepted:
- We present questionnaires, open-text, other self-reported data, and auxiliary information about the participants in the “CEPAV_data” spreadsheet24. The file includes participants’ ID, sex, age, height, weight, experimental conditions, and questionnaire responses (the “self_reports” sheet). To make it easier to use the database, we also included averages for physiological and behavioral data from selected moments of the study in the file, which were used for the Summary of the Physiological, Affective, and Behavioral Activity During the Competitive Esports (Technical Validation section) and presented in Fig. 5 (the “physio_behav” sheet)24.Uusberg, A. et al. Appraisal shifts during reappraisal. Emotion 23, 1985–2001 (2023).
- Participant movement was non-invasively tracked using three tri-axial accelerometers (model wGT3X-BT, Actigraph, USA), placed on the thighs (thigh left and thigh right; TL & TR) next to the knee and the right wrist (WR), allowing for the continuous observation of physical activity and gestures during gameplay. All accelerometers were initialized before the participant’s arrival to collect raw acceleration data at 30 Hz with the same start time using ActiLife software (version 6.13.4). We used the measure of the vector magnitude for the given accelerometer for each 1-second interval extracted with the ActiLife software.Behnke, M., Krzyżaniak, W., Nowak, J. et al. The competitive esports physiological, affective, and video dataset.
Sci Data 12, 56 (2025). https://doi.org/10.1038/s41597-024-04364-z - Article
PubMed
MATH
Google Scholar - Park, C. Y. et al. K-EmoCon: A multimodal sensor dataset for continuous emotion recognition in naturalistic conversations. Sci. Data 7, 293 (2020).Cohn, M. A., Fredrickson, B. L., Brown, S. L., Mikels, J. A. & Conway, A. M. Happiness unpacked: Positive emotions increase life satisfaction by building resilience. Emotion 9, 361–368 (2009).
- Prospective Studies Collaboration. Age-specific relevance of usual blood pressure to vascular mortality: A meta-analysis of individual data for one million adults in 61 prospective studies. The Lancet 360, 1903–1913 (2002).Gualano, M. R., Lo Moro, G., Voglino, G., Bert, F. & Siliquini, R. Effects of Covid-19 lockdown on mental health and sleep disturbances in Italy. Int. J. Environ. Res. Public Health 17, 4779 (2020).
- Katsigiannis, S. & Ramzan, N. DREAMER: A database for emotion recognition through EEG and ECG signals from wireless low-cost off-the-shelf devices. IEEE J. Biomed. Health Inform. 22, 98–107 (2017).Goldberg, D. P. & Hillier, V. F. A scaled version of the general health questionnaire. Psychol. Med. 9, 139–145 (1979).
- Human-Technology Interaction, Eindhoven University of Technology, Eindhoven, The NetherlandsLawes, M., Hetschko, C., Schöb, R., Stephan, G. & Eid, M. The impact of unemployment on cognitive, affective, and eudaimonic well-being facets: Investigating immediate effects and short-term adaptation. J. Pers. Soc. Psychol. 124, 659–681 (2023).
- Participants’ upper bodies were continuously captured on video using an HD camera positioned in between the monitors, utilizing the Open Broadcaster Software25. The camera was set approximately 65 cm away from the participants’ heads, with a recording at 30 FPS. We also captured the back view of the experimental view with the camera near the ceiling (Fig. 2). These recordings were primarily used to monitor the study’s progress and ensure participant safety.Medland, H., De France, K., Hollenstein, T., Mussoff, D. & Koval, P. Regulating emotion systems in everyday life: Reliability and validity of the RESS-EMA scale. Eur. J. Psychol. Assess. 36, 437 (2020).
- Article
Google Scholar
Affective science is an interdisciplinary field that draws upon methods and findings from psychology, cognitive science, neuroscience, computer science, biology, and other related fields to understand the complexities of affective phenomena and to determine how they influence human behavior. Multimodal data are needed to thoroughly investigate affective phenomena. Different scientific backgrounds equip researchers with unique skills for data collection. For example, psychologists excel in experimental designs, computer scientists excel in data mining from digital platforms, and biomedical researchers excel in collecting biological samples. Interdisciplinary teams leverage these diverse methodologies to approach research questions by collecting comprehensive multi-modal datasets. - Diener, E., Emmons, R. A., Larsen, R. J. & Griffin, S. The Satisfaction with Life Scale. J. Pers. Assess. 49, 71–75 (1985).Article
Google Scholar - The physiological data quality was assured by following recommendations in affective science33. First, the data were collected by experimenters who completed at least 30 hours of training in psychophysiological research provided by MB. Second, prior to performing preprocessing, the first author (MB) visually inspected all physiological signals. Before inclusion in the database, MB manually double-checked all datasets for missing or corrupted data.
Reprints and permissionsPanzeri, A. et al. Factors impacting resilience as a result of exposure to COVID-19: The ecological resilience model. PLoS One 16, e0256041 (2021).
Google Scholar
Article
PubMed
PubMed Central
Google Scholar
MATH
Google Scholar
Majka, E. A., Guenther, M. F. & Raimondi, S. L. Science bootcamp goes virtual: A compressed, interdisciplinary online CURE promotes psychosocial gains in STEM transfer students. J. Microbiol. Biol. Educ. 22, 10–1128 (2021).
CAS
PubMed
MATH
Google Scholar
PubMed Google ScholarArticle
MATH
Google Scholar
MATH
Google Scholar
PubMed Google ScholarPreece, D. A. et al. The Perth Alexithymia Questionnaire-Short form (PAQ-S): A 6-item measure of alexithymia. J. Affect. Disord. 325, 493–501 (2023).
PubMed
PubMed Central
MATH
Google Scholar
Article
MATH
Google Scholar
Google Scholar
Article
PubMed
PubMed Central
Google Scholar
PubMed
PubMed Central
MATH
Google Scholar
Ranganathan, H., Chakraborty, S. & Panchanathan, S. Multimodal emotion recognition using deep learning architectures. In Winter Conf. Appl. Comput. Vision (2016).
Google Scholar
Article
CAS
PubMed
MATH
Google Scholar
PubMed
PubMed Central
MATH
Google Scholar
Article
CAS
PubMed
MATH
Google Scholar
Google Scholar
Article
MATH
Google Scholar
Google Scholar
PubMed
MATH
Google Scholar
PubMed
Google Scholar
PubMed
Google Scholar
Fingerman, K. L. et al. Living alone during COVID-19: Social contact and emotional well-being among older adults. J. Gerontol. B 76, e116–e121 (2021).
Google Scholar
Gross, J. J. & John, O. P. Individual differences in two emotion regulation processes: Implications for affect, relationships, and well-being. J. Pers. Soc. Psychol. 85, 348–362 (2003).
MATH
Google Scholar
Article
PubMed
PubMed Central
MATH
Google Scholar
PubMed
PubMed Central
Google Scholar
CAS
MATH
Google Scholar
CAS
PubMed
PubMed Central
Google Scholar
PubMed
Google Scholar
Steger, M. F., Frazier, P., Oishi, S. & Kaler, M. The meaning in life questionnaire. J. Couns. Psychol. 53, 80–93 (2015).
PubMed
PubMed Central
Google Scholar
Becerra, R., Preece, D. A. & Gross, J. J. Assessing beliefs about emotions: Development and validation of the Emotion Beliefs Questionnaire. PLoS One 15, e0231395 (2020).
PubMed
MATH
Google Scholar
The sample consisted of 300 male players of Counter-Strike: Global Offensive (CS: GO), aged between 18 and 32 years, with a mean age of 21.95 years (SD = 2.29). Competitive experience varied within the group: 200 players (67%) had no experience, 76 (25%) had competed in local tournaments, 17 (6%) had participated nationally, and six (2%) had taken part in international competitions. One participant did not disclose his competitive background. Esports provided an additional income source for 17 participants, while the rest did not earn money through gaming. On average, participants had been playing CS: GO for 9.13 years (SD = 5.22), with a mean total gameplay time of 2225.69 hours (SD = 1980.55) as recorded in their Steam Library (Valve Corp., SA). The number of participants varied across CS: GO ranks, with 9 participants ranked as Silver I, 2 as Silver III, 7 as Silver IV, 4 as Silver Elite, 4 as Silver Elite Master, 10 as Gold Nova I, 14 as Gold Nova II, 12 as Gold Nova III, 11 as Gold Nova Master, 28 as Master Guardian I, 21 as Master Guardian II, 26 as Master Guardian Elite, 27 as Distinguished Master Guardian, 29 as Legendary Eagle, 32 as Legendary Eagle Master, 19 as Supreme Master First Class, 44 as Global Elite. Details related to inclusion and exclusion criteria and the process of sample size determination are described elsewhere6.
PubMed Google Scholar
ADS
CAS
PubMed
PubMed Central
MATH
Google Scholar
Project and Match Procedures. The red frames represent a procedure for all performances (to simplify the figure, we depicted it in detail only for baseline performance), namely prematch physiology, affective experience, Counter-Strike: Global Offensive match, and recovery. Baseline and post-intervention questionnaires include negative prior mindsets, positive and negative affective experiences, affect regulation strategies, well-being, ill-being, alexithymia, and emotion belief measures. Affective self-report includes affective experience and demands and resources evaluation. Emotion recall tasks include recalling and describing situations from the tournament that elicited positive and negative affective experiences and evaluating them using affective experience, situational appraisals and affect regulation strategies measures. One month after Stage 3, participants were asked to fill in follow-up questionnaires, the same set as at baseline and post-intervention. Figure reproduced from our previous article6, used under a CC BY license.
Google Scholar
MATH
Google Scholar
The calculated SNR indicated the high quality of all collected signals35, with mean SNR ranging from 21.90 dB to 68.05 dB depending on the physiological signal and study. We identified outliers with the median absolute deviation, with a cutoff of 3, as recommended by Leys et al.36,37, resulting in 416 signals (3.94% of all calculated SNR values) identified as SNR outliers. Next, the first author (MB) visually inspected all flagged data to determine whether the signal should be deleted and classified as an artifact, resulting in 17 signals being identified as artifacts. Outlying signals are marked with yellow color in the “SNR” sheet with additional comments from the first author on reasons that potentially influenced the outlying values (e.g., disconnecting ECG and ICG electrodes after the study while the signals were still being recorded).
PubMed Google ScholarTomaka, J., Blascovich, J., Kelsey, R. M. & Leitten, C. L. Subjective, physiological, and behavioral effects of threat and challenge appraisal. J. Pers. Soc. Psychol. 65, 248–260 (1993).
PubMed
PubMed Central
MATH
Google Scholar
PubMed
PubMed Central
Google Scholar
MATH
Google Scholar
PubMed
MATH
Google Scholar
MATH
Google Scholar
Google Scholar
Participants played Counter-Strike: Global Offensive (CS: GO) in a deathmatch mode on the Dust II map, competing against the highest difficulty level of computer-generated opponents (bots) without random weapons. All matches were structured into prematch baseline measurements (2 minutes), gaming (2 minutes), and recovery (2 minutes) (Fig. 1). During all match phases, we collected cardiovascular, behavioral, and video data. Before each match, participants also shared their affective experience and provided demands and resources evaluations.
PubMed
Google Scholar
PubMed
PubMed Central
MATH
Google Scholar
Google Scholar
Leys, C., Delacre, M., Mora, Y. L., Lakens, D. & Ley, C. How to classify, detect, and manage univariate and multivariate outliers, with emphasis on pre-registration. Int. Rev. Soc. Psychol. 32, (2019).
PubMed
Google Scholar
Sibley, C. G. et al. Effects of the COVID-19 pandemic and nationwide lockdown on trust, attitudes toward government, and well-being. Am. Psychol. 75, 618–630 (2020).
Acknowledgements
Author information
Authors and Affiliations
Contributions
Sample size: 300 participants.
Corresponding author
Klussman, K., Lindeman, M. I. H., Nichols, A. L. & Langer, J. Fostering stress resilience among business students: The role of stress mindset and self-connection. Psychol. Rep. 124, 1462–1480 (2021).
Ethics declarations
Competing interests
Eysenck, H. J. & Eysenck, S. B. G. Manual of the Eysenck Personality Questionnaire (Junior & Adult). Hodder and Stoughton Educational (1975).
Additional information
Rights and permissions
Article
PubMed
MATH
Google Scholar
About this article
Cite this article
MATH
Google Scholar
Google Scholar
- Here, we present the Competitive Esports Physiological, Affective, and Video (CEPAV) dataset1. Esports represents a rapidly growing field in which well-trained individuals – gamers – compete using video games. In esports, gamers compete while seated in front of a screen, creating an ideal environment to study affective responses, including emotional experiences and real-time cardiovascular reactions to performance2,3,4,5. This setting allows for the examination of high-stakes performance with continuous real-time monitoring of affective responses at multiple levels. Using esports as a model allowed us to gain insights into the interplay between emotional states and physiological responses during intense gameplay sessions.
- Behnke, M., Gross, J. J. & Kaczmarek, L. D. The role of emotions in esports performance. Emotion 22, 1059–1070 (2022).
- Benet-Martínez, V. & John, O. P. Los Cinco Grandes across cultures and ethnic groups: Multitrait-multimethod analyses of the Big Five in Spanish and English. J. Pers. Soc. Psychol. 75, 729–750 (1998).
- Article
PubMed
MATH
Google Scholar